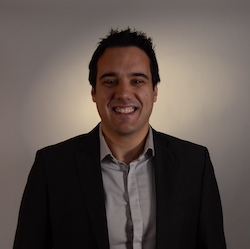
Hi! I’m Germain Salvato Vallverdu, associate professor at the Université de Pau et des Pays de l’Adour, specialist in theoretical chemistry, molecular modeling and numerical simulations at IPREM institute.
Have a look at my research projects or softwares I develop or contribute to. See more general topics on my webpage.